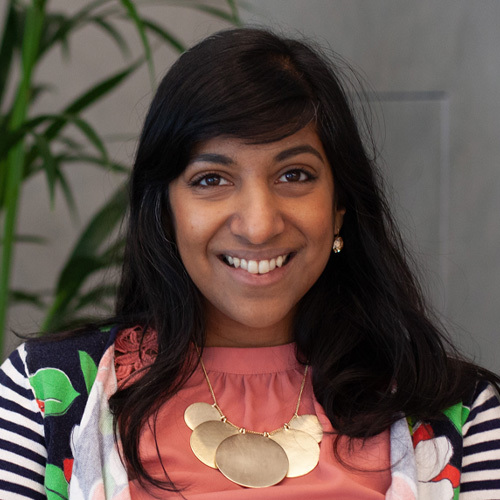
Inferring causal networks from the correlated tangle of gene expression data to paint detailed molecular descriptions of disease mechanisms.
Is it possible for two individuals to be friends with the same groups of people, but have nothing to do with each other? Be it ‘unfriended’ contacts on Facebook, or long-time colleagues who have never once emailed each other let alone worked on the same project, the evidence is there that two people can remain strangers despite having a large overlapping social network. This is true too of other kinds of interactions beyond social relationships. In professional heavyweight boxing, for example, the Klitschko brothers famously fought alongside each other in the same era but, in keeping with a promise they made to their mother, never against each other.
One such interaction we care a lot about in drug discovery is the regulatory relationships between genes. Untangling these causal networks from the correlated mess of gene expression data allows us to paint detailed molecular descriptions of disease mechanisms. And crucially, being able to separate causal and direct gene regulation (fig 1C) from the confounding transcriptional co-regulation of genes (fig 1A) and indirect regulation (fig 1B) means one can more accurately identify the target genes for therapeutic drug interventions.
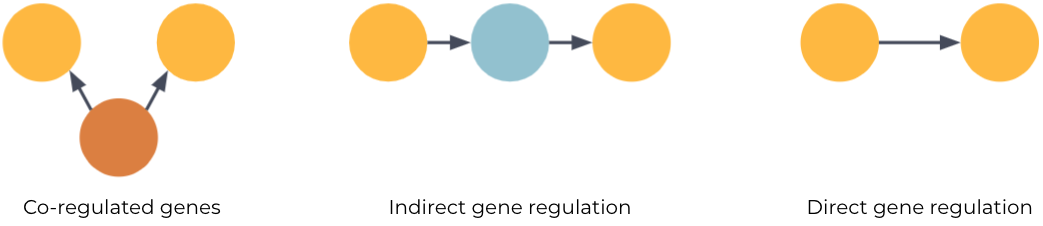
If we imagine a network where each entity is a node in the graph and relations are edges then the observation is the following: Two entities can be semantically similar, i.e. with large overlapping neighbourhoods, without necessarily being causally linked.
Despite the alluring activity of, say, performing arithmetic on vector representations of word entities, the constraints of Euclidean geometry itself are obstacles to fully expressive representations. One idea that has gained significant traction in recent years is the use of more general Riemannian manifold embeddings to encode for certain graph features missed by flat Euclidean space. The key development was the introduction of hyperbolic manifold embeddings, which demonstrated how the geometry can be exploited to uncover latent graph features, in this case the hierarchy of nodes in the graph. Despite their successes, these curved manifolds are ultimately still metric spaces and will struggle with the specific point of disambiguating semantic similarities from causal relations. In our paper we show how we can treat this feature as an inductive bias in the design of more representative graph embedding models. We introduce the following three ideas.
The first is to consider pseudo-Riemannian manifolds, such as spacetime manifolds from physics. The central point is that we can partition our manifold, with respect to a given entity, into spacelike and timelike regions, depending on the sign of the squared displacement. This is illustrated in Figure 2 for flat Minkowski spacetime. We see an arrangement where two entities in a directed graph can be spacelike separated (i.e. not causally related) and yet have an arbitrarily large overlapping sets of predecessors and successor nodes.
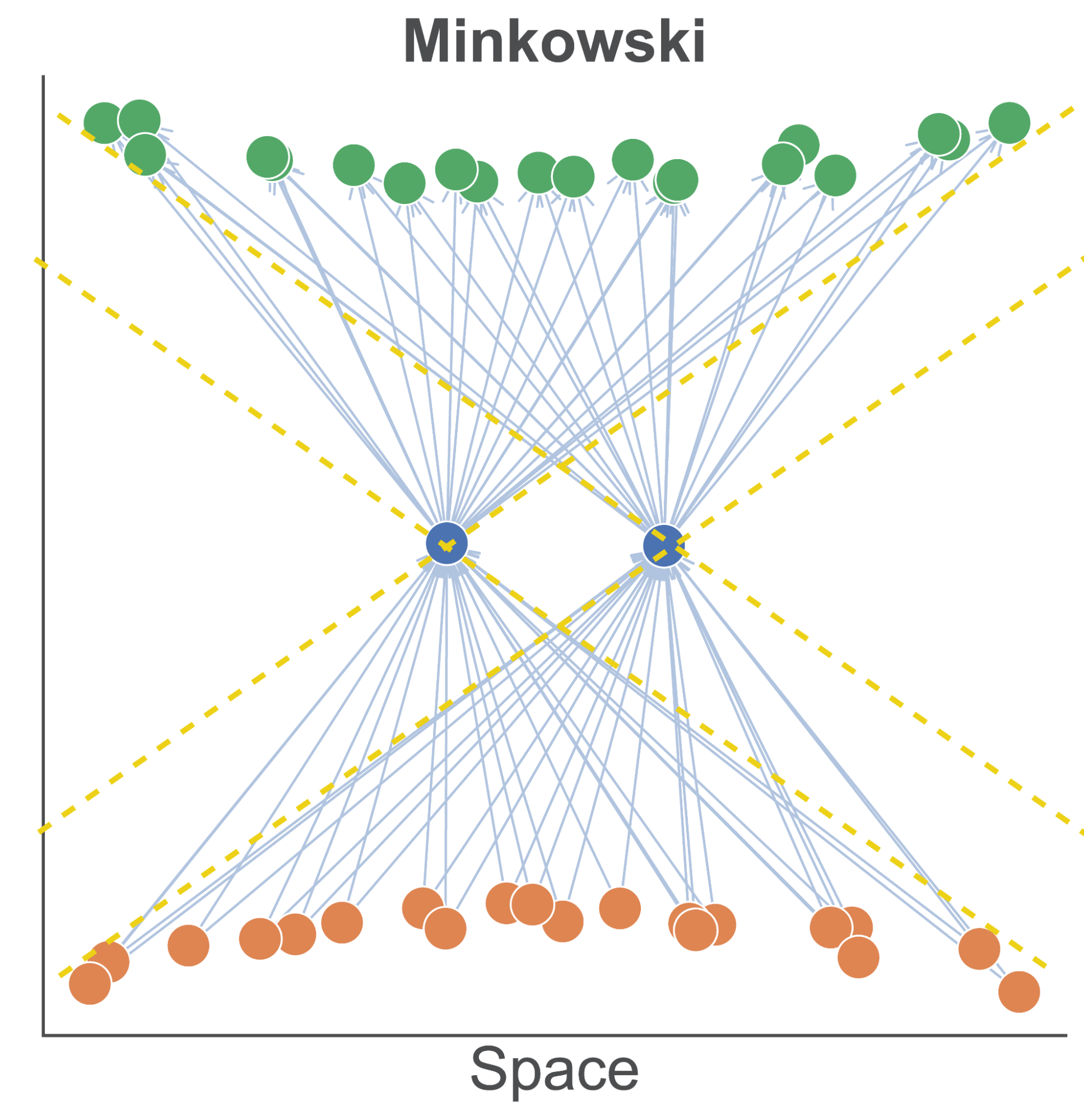
The second idea is to design a model that accommodates both direct (transitive) and indirect causal relationships via a novel loss function that explicitly breaks the time symmetry and introduces a probability that decays into the far future. Finally the third idea is to consider manifolds with non-trivial topologies to model graphs with cycles. One example we consider in the paper is anti-de Sitter spacetime, which is topologically equivalent to a higher-dimensional cylinder with a closed time dimension.
Together, these unique features allow us to go beyond the representational capacity of standard Euclidean and Hyperbolic geometries, to model unique graph architectures that have real world significance, in biology and boxing alike. This avenue of research also opens the door to important future work, including network inference, or the extraction of a causal graph structure from gene expression data, which has important applications in drug discovery. We hope that applications of this novel method in biology bring us one step closer to discovering life-changing medicines with a higher likelihood of clinical success.
Back to blog post and videos