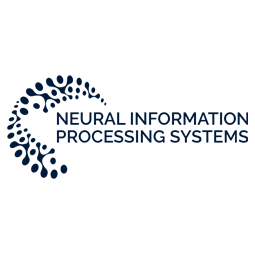
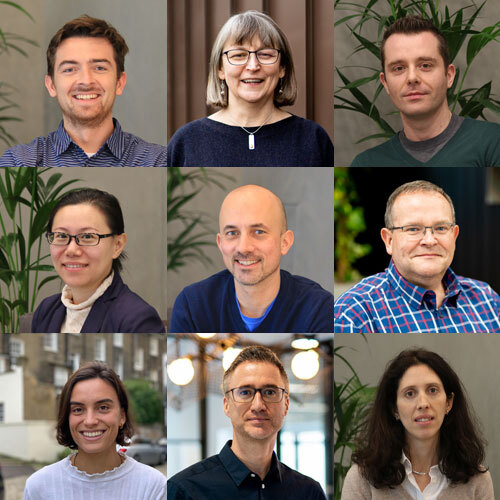
Join the BenevolentAI team at the NeurIPS 2021 LMLR workshop.
Neural Information Processing Systems (NeurIPS) is a prestigious peer reviewed conference that aims at publishing cutting edge research in artificial intelligence, machine learning and their related areas.
We look forward to returning to NeurIPS to present two posters at the LMRL workshop.
Poster 1: Contrastive VAE models to identify independent disease mechanisms in single-cell data
Identifying differences between sample groups such as patient cohorts or cell types provides insight into characteristics particular to a group of interest. Contrastive latent variable models can summarize signals in the data into a low-dimensional space and yield background and foreground specific latent variables. However, the sources of variation in the latent space can be entangled, and formulating VAE models that encourage independence on latents is an open area of research.
In this work, we focused on scRNA-seq data assumed to have underlying background variation shared among all the samples and additional foreground signals specific to some. Our task was to separate the signals into background and foreground-specific independent latents. We investigated several contrastive VAE models for scRNA-seq data and observed that combining them with Independent Component Analysis (ICA) leads to a good separation of independent background and foreground components, thus encouraging contrastiveness as well as independence. Further work on such models will allow us to better understand disease biology and lead to the discovery of novel drug targets and specific biomarkers.
Authors: Atanasiu Demian, Harry Rose, Sam Abujudeh & Meltem Gürel
Presented by: Atanasiu Demian - BenevolentAI Summer Intern.
Atanasiu completed an MSci in Mathematics & Statistics at University of Bristol and is now in the third year of his PhD in Medical Sciences at University of Oxford. Atanasiu’s research focuses on Bayesian modelling of single cell data, in particular, applications of deep learning. Atanasiu is looking to apply this research to better understand the production of hematopoietic stem cells in embryos.
Poster 2: Benchmarking algorithms to identify clinically-relevant sub-populations of patients
A key focus of precision medicine initiatives is to move beyond the ‘one-size-fits-all’ treatment approach by identifying sub-populations of patients with different clinico-pathological manifestations of disease or response to standard-of-care treatments. Patients’ sub-populations can then be further characterized to identify underlying biological mechanisms driving the specific clinical phenotype, enabling personalised prioritization of therapeutics.
In this work, we sought to benchmark the application of different combinations of feature transformations with patients clustering algorithms. We assessed performances by quantifying how well these algorithms recovered known clinically-relevant sub-populations and how stable the results are in both simulated and real-world patient-level transcriptomic datasets, including multiple oncological diseases.
When benchmarking algorithms across multiple use-cases, we observed consistent performance patterns and identified methods with slightly better recovery of patients’ sub-populations. These results pave the way for identifying sub-populations of patients with mechanism-specific dysregulations (endotyping) by applying selected algorithms on features specifically associated with a pathway of interest rather than the whole feature space.
Authors: Manuela Salvucci, Meltem Gürel, Samer Abujudeh, Marika Catapano, Gregor Lueg, Matyas Korom, Manav Leslie, Peter McErlean, Francesca Mulas
Presented by: Manuela Salvucci
Manuela has joined BenevolentAI as a senior Bioinformatics Data Scientist in the PMPT Precision Medicine team. She studied biomedical engineering, completed a PhD in systems biology and continued
Back to events